The research in AI Lab is motivated by the challenge that all transportation agencies are currently facing and will continue to face: The conflict among the increasing demands on transportation systems, the continually aging transportation infrastructures, and the stringent labor and financial resources. Such a challenge bears an urgent, yet long-lasting need for an intelligent way to construct a reliable and cost-effective transportation infrastructure system.
Sensing-Based Transportation Asset Management
Transportation assets are the most critical element in transportation systems to provide critical functional support for road users. Public agencies need to make optimized decisions to maintain the functionality of their transportation assets while minimizing costs. These optimized decisions can and will be relying on a comprehensive synthesis using sensing-based systems. Therefore, AI Lab envisions its effort in this direction on developing fundamental computer vision and sensing signal processing algorithms to construct a system that can intelligently evaluate, monitor, and diagnose transportation asset conditions.
- Ai, C. (PI), and Tessier, R. “Development of a Salt Spreader Controller Program that Correlates its Material Dispensation Rate to Machine “Sensed” Roadway Weather Parameters.” Massachusetts Department of Transportation. 2022-2024.
- Ai, C. (PI). “Automated Guardrail Inventory and Condition Evaluation.”Massachusetts Department of Transportation. 2021-2022.
- Ai, C. (PI). “A Method for Pavement Marking Inventory and Retroreflectivity Condition Assessment Using Mobile LiDAR.” Massachusetts Department of Transportation. 2020-2022. [Webinar].
- Christofa, E. (PI). and Ai, C. (co-PI). “Effectiveness of Bicycle Boxes in Massachusetts.” Massachusetts Department of Transportation. 2020-2021.
- Ai, C. (PI). “Improving Pedestrian Facility Inventory Data in Massachusetts using Mobile Light Detection and Ranging (LiDAR).” Massachusetts Department of Transportation. 2018-1019.
Sensing-Based Transportation Safety Awareness
AI Lab sees great opportunities in the research areas of transportation safety awareness by employing sensing technologies. Sensing technologies have inherent advantages to answer some of the fundamental questions in this research area, e.g. how to accurately and objectively acquire and evaluate safety measures, how to proactively be aware (“predict”) of the potential safety issues, etc. However, naturally, many research issues in transportation safety may not be instantly resolved by simply applying sensing technologies. For example, it may remain challenging to quantify and estimate human perception and psychological behavior. Therefore, AI Lab envisions the research in this direction focusing on these challenging issues, by integrating the traditional safety analysis methods, e.g., driving simulation, and survey, etc., and the sensing-based methods, e.g., eye tracking, vehicle trajectory analysis, etc.
- Xie, Y. (PI), Ai, C.(co-PI), and Liu, B. (co-PI). “Uncovering the Root Causes of Truck Rollover Crashes on Ramps.”Massachusetts Department of Transportation. [Webinar].
- Ai, C. (PI), with Knodler, M. “Collecting Model Inventory Road Element (MIRE) Fundamental Data Elements (FDEs) for Intersections in Massachusetts.” Massachusetts Department of Transportation. 2018-2021.
- Ai, C. (PI), with Fitzpatrick, C. “Deflection Angle Effect on Continuous Driver Performance along Horizontal Curves.” U.S. Department of Transportation through the Safety Research Using Simulation (SAFER-Sim) University Transportation Center. 2020-2021.
- Ai, C. (PI), with Knodler, M. “Quantifying the Impacts of Situational Visual Clutter on Driving Performance Using Video Analysis and Eye Tracking.” U.S. Department of Transportation through the Safety Research Using Simulation (SAFER-Sim) University Transportation Center. 2019-2020. [Webinar]
Sensing-Based Infrastructure Preservation and Maintenance
To construct and maintain the most invested infrastructure in the whole transportation infrastructure system, studies on pavement and bridge condition evaluation and preservation have been actively conducted during the past decades. Until recently, the emerging 3-D scanning laser technology has revolutionized both the industry’s and public transportation agencies’ practices, especially in the area of data acquisition. However, while the overwhelmingly large amount of data provides unprecedented details for pavements and bridges, it has become increasingly challenging to accurately and efficiently extract useful information supporting maintenance decisions. Therefore, AI Lab envisions the research in this direction focusing on bridging the gap between the “raw data” and the “derived knowledge” of the infrastructure condition, through harnessing exciting collaborations in the areas of image processing and signal processing, structure analysis, deterioration modeling, infrastructure management system, etc.
- Gerasimidis, S. (PI), Breña, S. (co-pI), and Ai, C. (co-PI). “Development of Improved Inspection Techniques Using LiDAR for Deteriorated Steel Beam Ends.” Massachusetts Department of Transportation. 2022-2024.
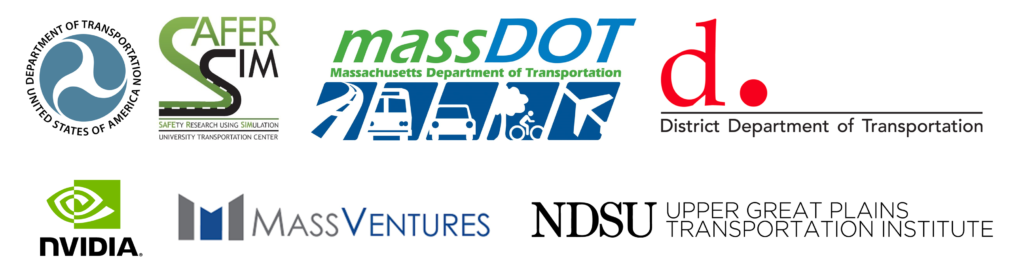